Genetics
When The Student Is A Computer: Teaching Machines To Do New Tricks
October 29, 2018
The combination of machines, people and data is sparking a scientific revolution in genomics
By: Jeffrey Reid, PhD, Vice President, Head Of Genome Informatics, Regeneron Genetics Center
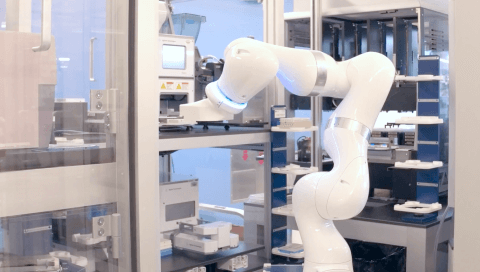
When artificial intelligence (AI) or machine learning (ML) makes the news, the focus tends to be flashy applications such as self-driving cars, social media bots, and face recognition. The very names of these methods seem designed to leave the impression that we are moving into a sci-fi future where computers are thinking and learning just like us, and may be becoming something more human than human.
But clear away the hype, and most AI/ML methods are narrowly focused—just fancy names for accurate, lightning-fast computational pattern recognition. In fact, some of these methods are not even new or innovative, just old-fashioned data analysis with improved branding. Unfortunately, the fancy name evoking the alluring idea of computers thinking like humans obscures the fact that the methods themselves aren’t nearly as important as the data from which they learn.
“…we are building data sets that can turbo-charge AI/ML strategy to speed up our ability to pull insights out of Electronic Health Records (EHR) and genetic data.”
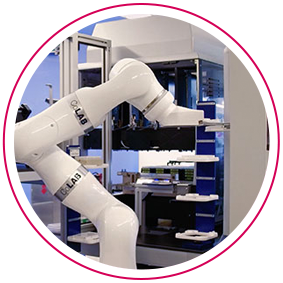
One of the Regeneron Genetics Center's robotic employees hard at work preparing lab samples
This is particularly true in biology, medicine, and pharmaceutical development, as biology is extremely complex, medical record data is usually very messy, and our understanding of the impact of genomic variation is still in its infancy. At the Regeneron Genetics Center® (RGC), we’re trying to improve this by pairing AI/ML technology with some of the world’s best, most inquisitive scientists and an unprecedented amount of robust genetic and real-world health data. The combination of machines, people, and data is sparking a scientific revolution in genomics, the field in which computational and statistical techniques are applied to derive biological insights from genome sequence and human trait data. Bringing the data, tools, and people together like this is helping us model diseases and look for new drug targets and therapeutic indications.
For example, here at the RGC, we’re using the power of AI/ML tools to find the root causes of diseases like eczema and asthma and identify the very specific proteins needed to correct them.
Building tomorrow today
Through our partnerships with the Geisinger Health System, UK Biobank, and others, we are building data sets that can turbo-charge AI/ML strategy to speed up our ability to pull insights out of electronic health records (EHR) and genetic data. For instance, with the clinical data from the EHRs, we have worked hard on both manual and automated approaches to harmonize the information; so even though we may receive two very different types of data in different formats from different sources, once they are processed through our system we can make “apples-to-apples” comparisons. As a result, we can analyze the clinical and genetic data to identify genetic variants and their impact on human biology—in turn, validating current investigational therapies or leading to new areas of therapeutic research.
“Bringing the data, tools, and people together like this is helping us model diseases and look for new drug targets and therapeutic indications.”
Along the way, we’ve created one of the world’s most comprehensive genetics databases. This database of rich information lays the foundation for scientific research that will lead to large-scale, life-changing discoveries. But these discoveries can’t be made by machine alone—it’s truly a team approach that relies on people, data, and computation all working together toward the common goal of improving people’s lives with important new medicines.